CSSE team wins 2nd round of Coleridge Initiative Food for Thought Challenge
Published: Nov 3, 2022 7:40 AM
By Joe McAdory
Auburn Engineering is making a strong impression at the Coleridge Initiative Food for Thought Challenge.
The team of Shubhra Kanti “Santu” Karmaker, assistant professor in computer science and software engineering, and doctoral students Naman Bansal and Alex Knipper, in collaboration with Jingyi Zheng, assistant professor in the Department of Mathematics and Statistics, and Wenying Li, assistant professor in the Department of Agricultural Economics, took first place and $10,000 in the competition’s second interim challenge.
Auburn’s team, “Big Data,” won the competition’s first interim challenge and another $10,000 earlier this fall.
In association with the U.S. Department of Agriculture, 12 teams of researchers were selected to use natural language processing (NLP) and machine learning techniques to help the USDA’s domestic food and nutrition assistance programs link databases to develop a better understanding of Americans’ dietary habits.
This competition will provide the USDA with new ways to produce a crucial data resource: the Purchase to Plate Crosswalk (PPC), which combines retail scanner data from Information Resources, Inc. (IRI) food item transactions with the USDA Agricultural Research Service (ARS)’ Food and Nutrient Database for Dietary Studies (FNDDS) nutrition information. The PPC crosswalk provides agencies with the ability to measure Americans’ diet quality and assess USDA Food Plan costs.
For the interim challenge’s second round, Karmaker said the team used both random forests and neural networks to form a model predicting links between retail scanner data (IRI) and the Food and Nutrient Database for Dietary Studies (FDNSS). Random forests are collections of decision trees, while neural networks are complex networks of simpler pattern recognition units like perceptions, sigmoid functions, etc.
Karmaker’s team implemented both approaches and found their performance to be comparable with random forests achieving slightly higher performance.
In the third round, the team will focus more on hyper-parameter tuning, an essential part of optimizing machine learning algorithms, to fine-tune both models and see if further improvements in the performances are possible.
Auburn Engineering hopes to have a clean sweep of the Coleridge Initiative Food for Thought Challenge as the third and final round winners will be announced on Nov. 30.
Media Contact: , jem0040@auburn.edu, 334.844.3447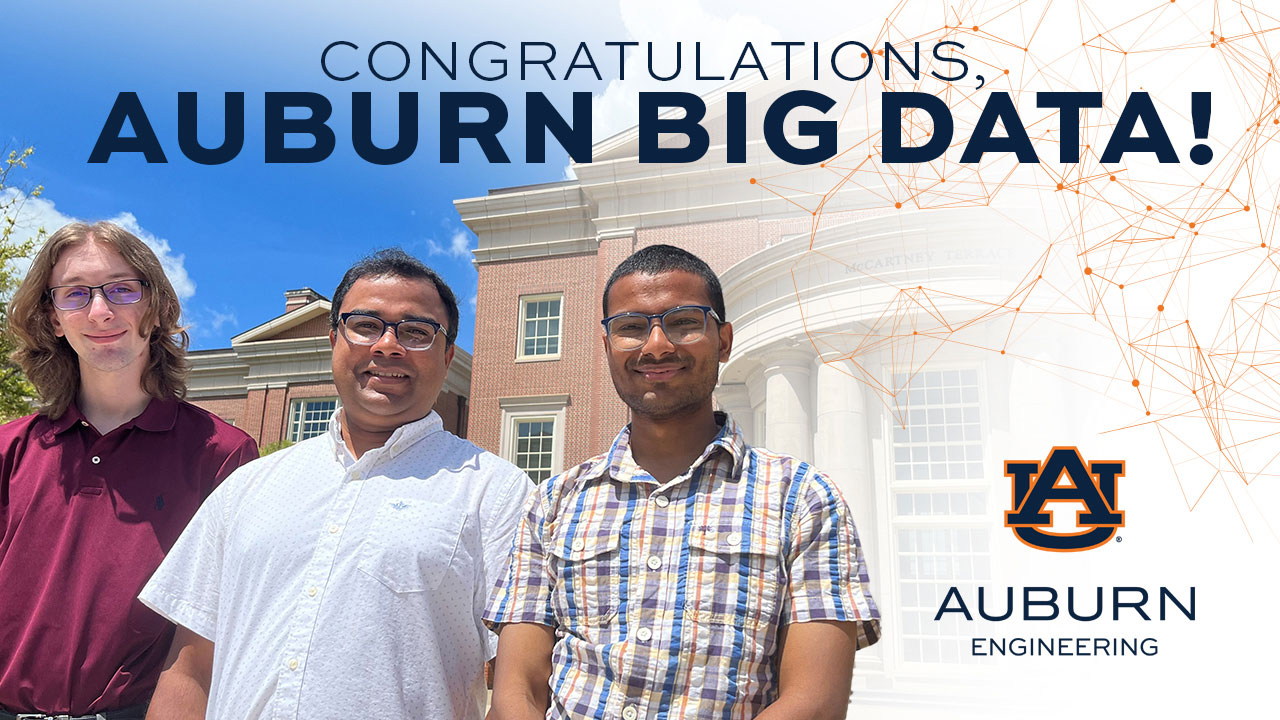
Researchers are using natural language processing and machine learning techniques to help the USDA’s domestic food and nutrition assistance programs link databases to develop a better understanding of Americans’ dietary habits.