Assistant professor in CSSE earns two grants that combine for $1.25 million
Published: Aug 2, 2023 9:50 AM
By Joe McAdory
Shubhra Kanti (Santu) Karmaker’s work is paying off, literally.
The assistant professor in computer science and software engineering recently secured grants combining nearly $1.25 million to: 1) develop cutting-edge Natural Language Processing (NLP) techniques for automatically analyzing and comparing multi-perspective narratives at scale and 2) create an innovative AI-assisted interactive learning environment for middle school science students as they conduct simulation-based experiments.
As principal investigator, Karmaker was recently awarded a two-year, $700,854 National Science Foundation (NSF) Research on Emerging Technologies for Teaching and Learning grant for his project, “An Intelligent Assistant to Support Teachers and Students in Simulation-Based Science Learning.”
Karmaker was awarded another $542,485 by the Air Force Office of Scientific Research (AFOSR) for his core NLP research, “A Novel Human-AI Collaborative Framework for Multi-Perspective Narrative Analytics and Braiding at Scale.”
“Receiving these grants is important because they provide researchers with the resources necessary to push forward,” said Karmaker. “Also, research awards offer credibility that your work is important within the academic community.”
Hari Narayanan, computer science and software engineering department chair, was excited for Karmaker.
“Utilization of artificial intelligence (AI) and natural language processing (NLP) continues to make an impact within industry, retail, and education,” said Narayanan, also a co-principal investigator on the NSF project. “That’s why pioneering research such as this is special because studies created today will shape technology tomorrow. Santu is growing into a respected researcher and will be pushing the boundaries of both fundamental and applied natural language processing research in the future.”
Karmaker’s AFOSR project is more theoretical and dedicated to the computational modeling and comparison of multi-perspective narratives (MPNs) describing an event of interest. MPNs are common across many domains, often emerging as historical perspectives, news stories, information gathered by detectives searching for a criminal, or hundreds of comments made by peer journal reviewers.
With an increase in the number of narratives to track and analyze, things can become intractable very quickly, and automation is desired.
“For any event that happens, most of us are not present there in real-time,” Karmaker said. “We see it from the narratives that people, or news organizations, bring to us. There are multiple perspectives. Interpretations are different. How do you assimilate all these alternative perspectives into a coherent story? Some people will hide part of it. Some add part of it. How do you make sense out of it all?”
Karmaker aims to address this problem by developing novel summarization algorithms to capture the commonalities (overlaps), differences (uniqueness), and conflicts among these MPNs. Through NLP and generative AI techniques, Karmaker will summarize alternative narratives with respect to the overlap-unique-conflict criteria.
“Given a particular sentence, we can convert information into numbers,” he said. “We call this a real-valued embedding vector. Computers don't understand text. They understand numbers. Once you translate semantics into numbers, then you can compute their similarities/differences easily. If they are very similar, then that is the common part. If they are not very similar, that is the unique part.”
Once the numbers from all sides are crunched – historical pieces, reviews, intelligence reports – common/unique/conflicting threads can be extracted, and a holistic yet concise perspective can be painted.
“Think about the intelligence community,” Karmaker said. “Intelligence-gathering happens all over the world. When you want to catch a terrorist, information is flowing fast in real time, but there is so much information that, a lot of time, human analysts miss them. If you have just one piece of information flowing in, that is not significant. But if you see repeated patterns within the information coming from many different places – “common themes” -- then we have a credible alert. Intelligence gathering in real-time is a big deal.”
Karmaker’s AFOSR project is expected to last for three years.
For the NSF project, Karmaker began developing an intelligent conversational agent (SimPal) that will first engage with teachers to solicit their instructional goals associated with simulation experiments and store these goals through a generic computational representation. Subsequently, SimPal will interact with students via a web browser as they run experiments. Students will be questioned, provided with real-time customized feedback, analyzed for progress, and provided with feedback opportunities. Responses will be passed back to the teacher. Karmaker will work closely with the Wisconsin Center for Education Research, which will test the AI platform in middle schools in Madison, Wisconsin.
The goals of this project are to provide:
- Access to high-quality learning materials anywhere;
- Adaptability to ensure students of all backgrounds/abilities receive customized feedback;
- Repeatability so students can run multiple experiments in a short time and receive real-time feedback;
- Scalability to reduce the burden of running inquiry-oriented science investigations.
“Scalability is important because what if there are 50 students per teacher and they are working on simulations?” Karmaker asked. “If the student has questions or difficulty, then the student must raise their hand and get immediate support from the teacher. That doesn’t scale very well. Also, students often work from home and need help while conducting experiments. Now, we have solutions that provide intelligent support in real time from anywhere.
“The question is… how do I know what the conversation should look like between the student and the AI agent? Many solutions have fixed, predefined conversational flows. It's not flexible. The best way would be to allow the teacher to define their own instructional objective, communicate that to the AI agent (SimPal) and have the agent help the student in the spirit of the teacher’s original instructional goal. Our agent will do exactly this. It will have a conversation with the teacher first. This is a novel part of our design. The second novel part is that the conversations with students will be guided by agent conversations with the teacher. Despite this conversational support, the student still will have some difficulty. In that case, the agent will gather and summarize the common difficulties and report back to the teacher.
“It will be a busy time working on these challenging projects in the upcoming two to three years, but making a positive research impact is important. Whether it’s creating new technologies to educate our children or building an AI agent to quickly assimilate abundant information, I’m happy to play a part.”
Media Contact: , jem0040@auburn.edu, 334.844.3447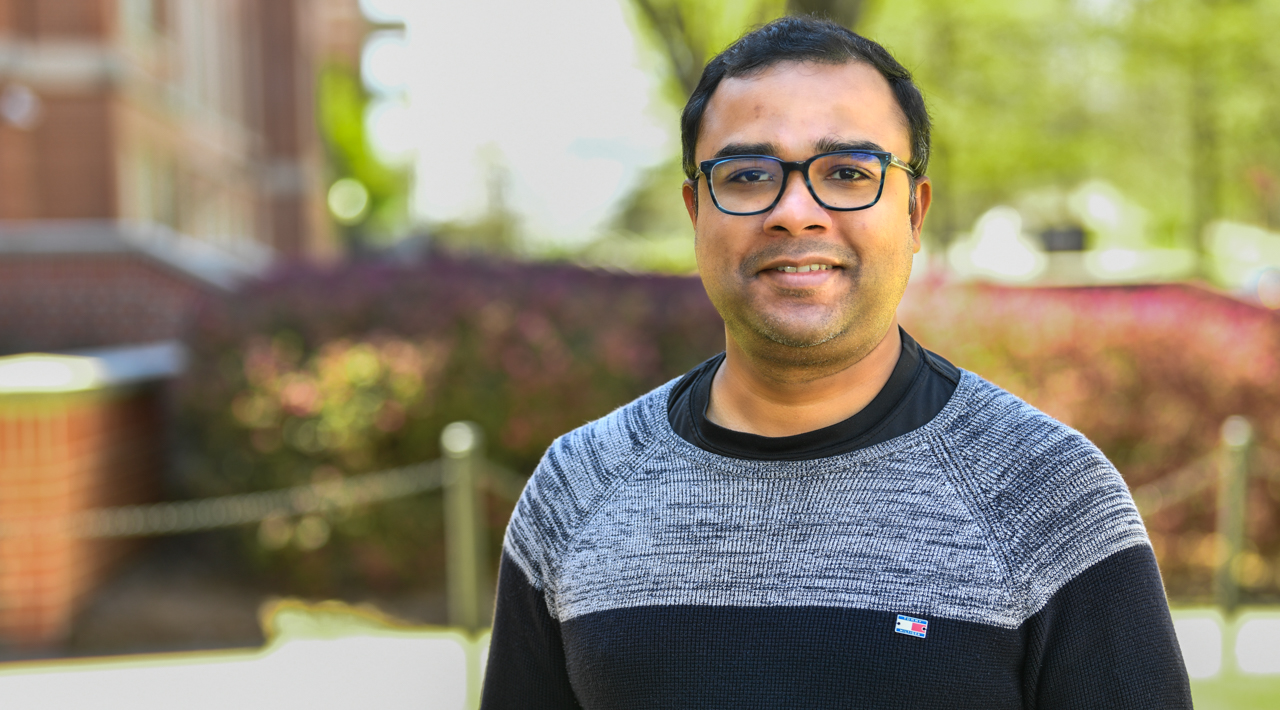
Shubhra Kanti "Santu" Karmaker said, "Whether it’s creating new technologies to educate our children or building an AI agent to quickly assimilate abundant information, I’m happy to play a part."