Research team from CSSE, COSAM and agriculture win USDA Coleridge Challenge
Published: Feb 8, 2023 12:20 PM
By Joe McAdory
After winning the first and second rounds of the Coleridge Initiative Food for Thought Challenge, a team of Auburn University faculty members and students from the colleges of engineering, agriculture, and sciences and mathematics, completed the sweep in December and won the third and final round.
The team of Shubra Kanti “Santu” Karmaker, assistant professor in computer science and software engineering and his doctoral students Naman Bansal and Alex Knipper, in collaboration with Jingyi Zheng, assistant professor in COSAM’s Department of Mathematics and Statistics, and Wenying Li, assistant professor in the Department of Agricultural Economics, earned $30,000 for winning the final round ($50,000 in total, combining all three rounds).
The challenge, in association with the U.S. Department of Agriculture, asked teams of data scientists to use natural language processing and machine learning to link food and nutrition databases (FNDDS) on a large scale.
“Competitions such as these provide students with an outstanding experiential learning opportunities and challenge them to determine which training model is best for a variety of machine learning tasks,” said Karmaker. “This isn’t just another homework assignment. This is real data that impacts lives. Learning more about shopping habits of consumers and providing this information to the USDA to help them track the food habits and nutrition status of the general mass is a great service.
“Auburn University was well represented in this very important competition. The team’s time dedication and commitment to accurate data modeling is a testament to the hard work of the faculty and the college’s rigorous computer science curriculum.”
Why was this study important? Scanner data derived from more than 120,000 households reveals consumer shopping habits, including when and where items were purchased, household demographics and health information. Purchase data can also be linked to product characteristics, such as the brand, which shows the type of products households prefer.
Over the past 10 years, the USDA has developed a larger data resource: the Purchase to Plate Crosswalk, which combines scanner data with the USDA Food and Nutrient Database for Dietary Studies. This crosswalk provides a comprehensive picture of the healthfulness of household purchases, allowing agencies to assess USDA Food Plan costs and measure the quality of Americans’ diets.
The goal of this competition is to provide the USDA with innovative ways to compile this crosswalk using natural language processing and machine learning.
For the final round, the team focused on hyper-parameter tuning, an essential part of optimizing machine learning algorithms, to fine-tune both tree-based and neural-network-based models and see if further improvements in the performances are possible. They spent more than 1,400 hours developing a model predicting links between scanner data and the USDA’s FNDDS.
Auburn Big Data used a simpler modeling approach, where simple statistical classifiers can be trained to predict the FNDDS label for a given IRI item. Random forest classification, a meta estimator that fits a number of decision tree classifiers on sub-samples of a dataset and uses averaging to improve predictive accuracy, was key in this approach. Simpler models are often quite robust and perform well for real-world data, Karmaker said.
“Our aim in this hyper-parameter tuning step is to see which of our already-implemented, easier-to-run downstream methods would better optimize the performance/efficiency tradeoff after having its training parameters optimized to the fullest,” Karmaker said. “This has resulted in a marginal increase in training time (20-30 minutes) and roughly a 5% increase in performance for our still-highest performing model, the random forest.”
Media Contact: , jem0040@auburn.edu, 334.844.3447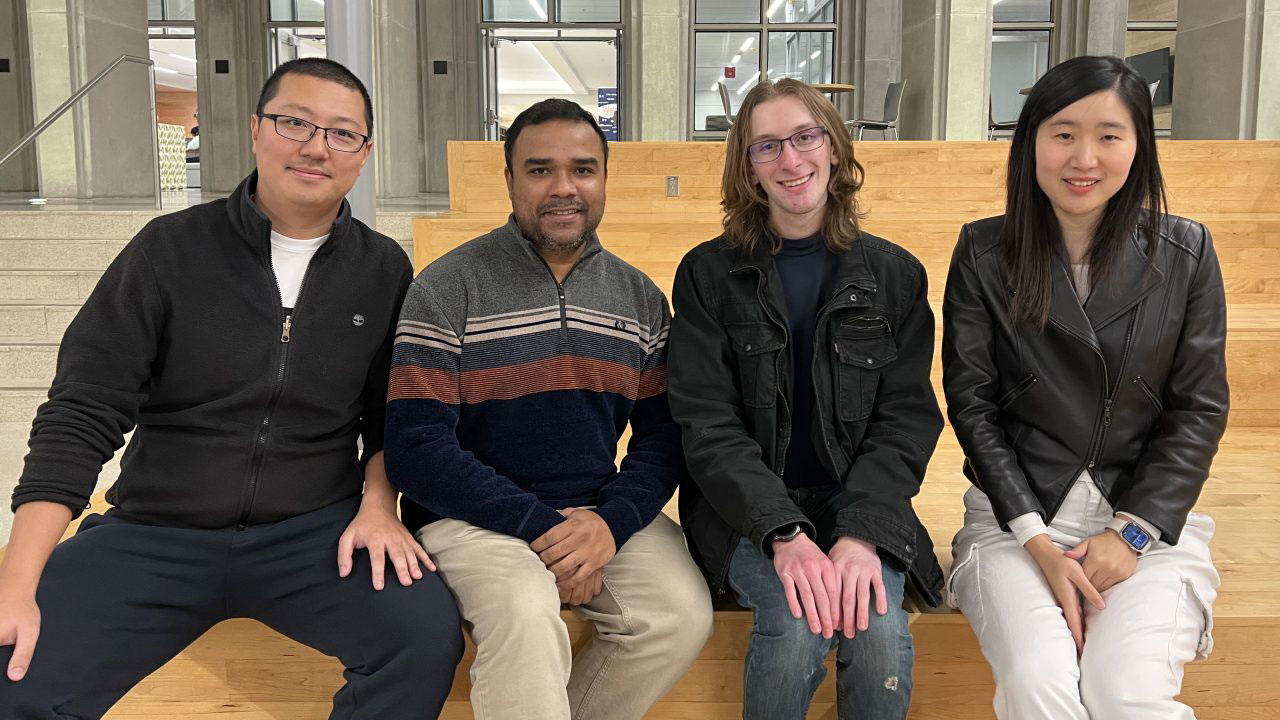
From left, Wenying Li (agricultural economics), Shubra Kanti Karmaker (computer science and software engineering), Alex Knipper (graduate student, computer science and software engineering), and Jingyi Zheng (COSAM).