Combating cancer subtype misdiagnoses with data science
Computer Science and Software Engineering
By Joe McAdory
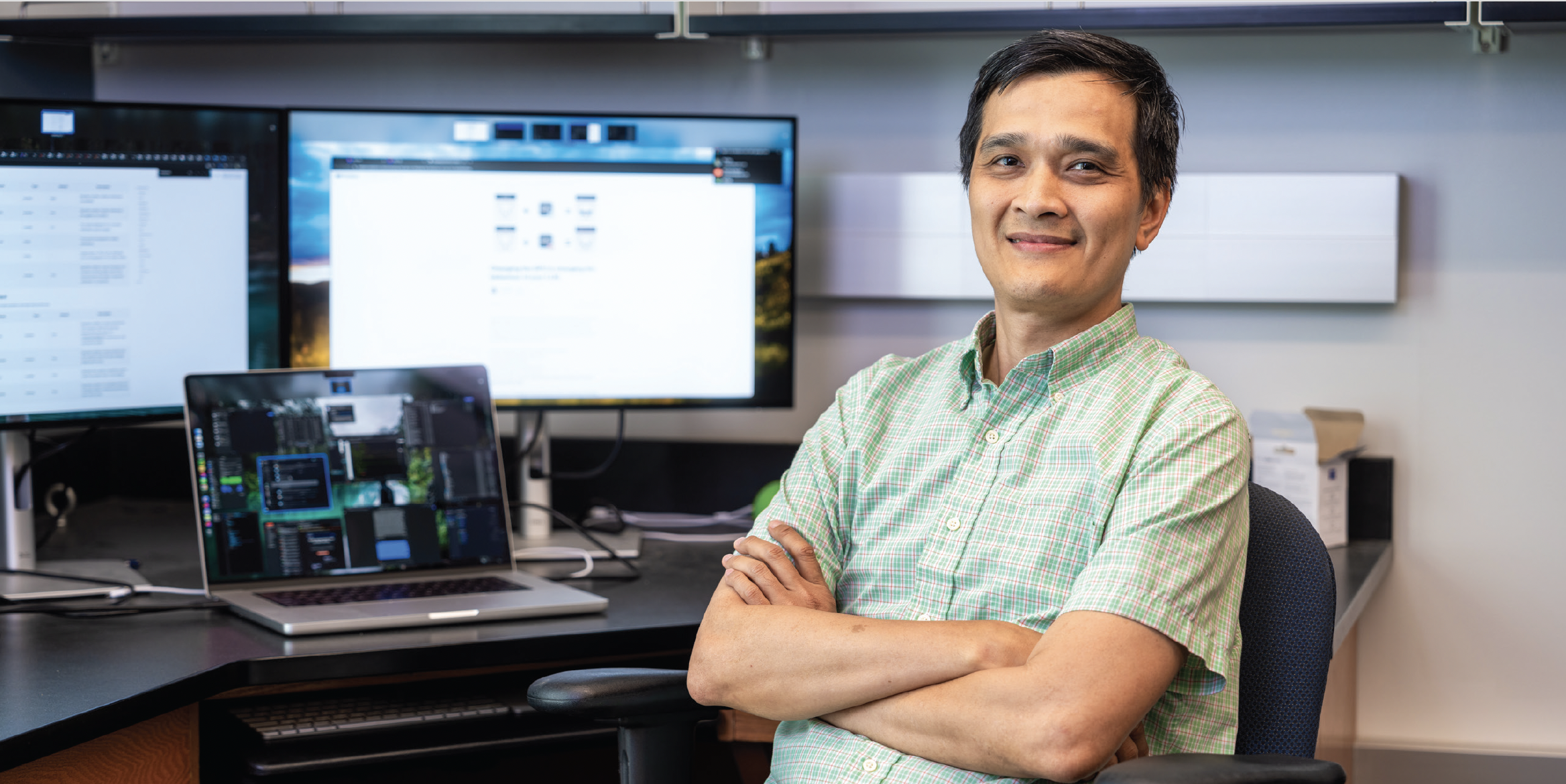
More than 40,000 patients across the nation could die each year as a result of misdiagnoses — with 37.8 percent of those related to cancer, a British Medical Journal study revealed. A diagnosis, followed by treatment, for one cancer subtype might not be suitable for another.
Tin Nguyen, however, has a proposal. Not with novel treatment methods or medicine. Not with laboratory testing that’s capable of targeting specific genes. And not with specialized, high-tech medical equipment. Instead, Nguyen prefers a computer. A big computer.
The outcome: fewer fatalities, less suffering and reduced health care costs. Nguyen earned a three-year, $1.2 million grant from the National Institutes of Health to find an ideal means of diagnosing cancer subtypes by means of data analysis — crunching years’ worth of laboratory discoveries and experiments and apply those to patients’ personalized pathway profiles. The result will create, among other deliverables, a complete analysis pipeline and web application able to integrate studies from multiple focuses.
“With computer science, you can collect data from multiple cancer-related experiments from the past decades and put them together,” said Nguyen, associate professor in the Department of Computer Science and Software Engineering and director of the Bioinformatics Lab at Auburn University.
“My solution is that we put data collected from these experiments into one analysis. That way we are not biased toward any study, and we are not biased toward any method or any type of molecular data. We can potentially have a complete picture. Then we can try to see treatments, or diseases, from many different aspects that one experimenter cannot do.”