Utilizing machine learning to improve data collection
Electrical and Computer Engineering
By Joe McAdory
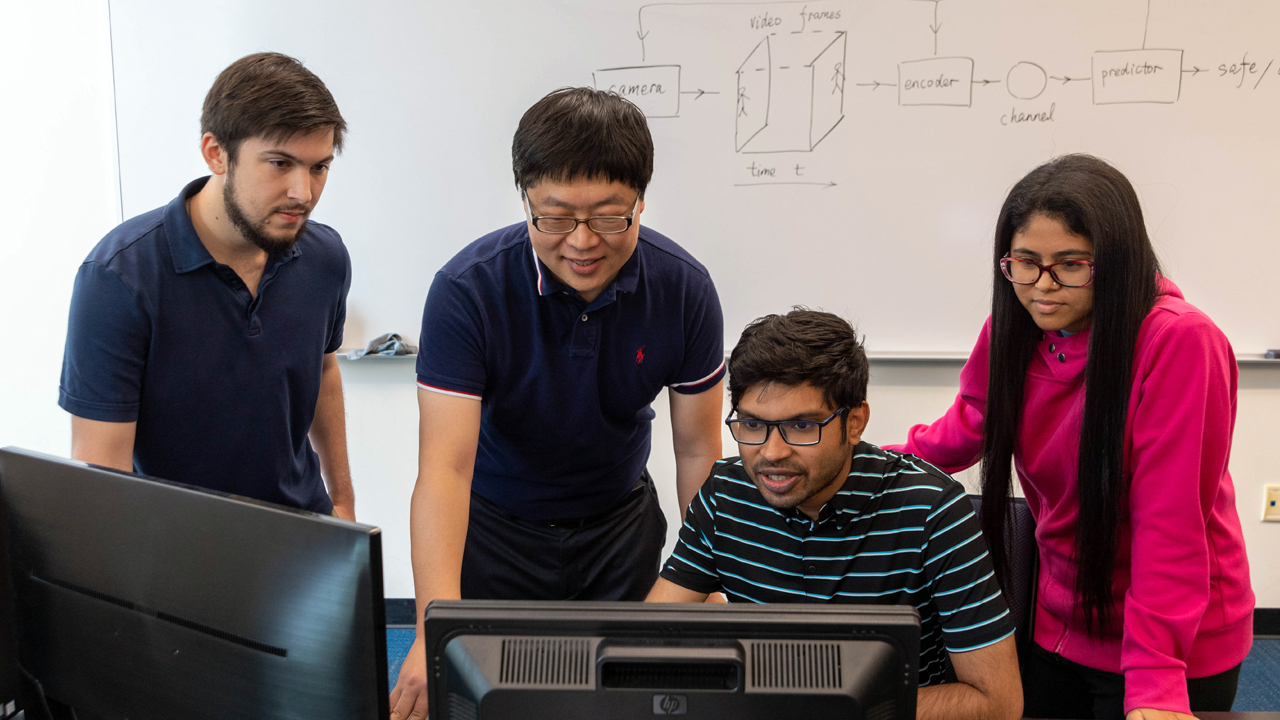
Fresh data is vital to operators because it is timely. Important data is vital to operators because it’s necessary for quick decision-making. Can machines learn to provide both sets of data simultaneously? Yin Sun, assistant professor in electrical and computer engineering, believe they can — and will prove it through his newest proposal, “Semantic and Goal-oriented Status Updating for Inference, Monitoring, and Decision-Making,” through a $516,000 National Science Foundation (NSF) CAREER Award.
“This is a very special and important recognition of my work,” Sun said. “Earning an NSF CAREER Award is great recognition for the university, the college, our department and will further inspire our team of graduate students.”
Sun becomes the department’s third CAREER Award winner in three years.
“As an administrator, it’s been special to watch Dr. Sun’s growth as a researcher and educator,” said Mark Nelms, Department of Electrical and Computer Engineering chair. “Dr. Sun is the embodiment of a scholar and educator who is not only committed to finding solutions to everyday technical problems.”
Sun’s proposal aims to use information-theoretic analyses and experimental studies to interpret how the freshness and semantics of information affect the performance of real-time inference, monitoring and decision-making systems; design new communications techniques and networking protocols for optimizing freshness/semantics measures and system performance; and explore the benefits of signal-aware status updating that goes beyond Age of Information to additional performance gain.
Researchers